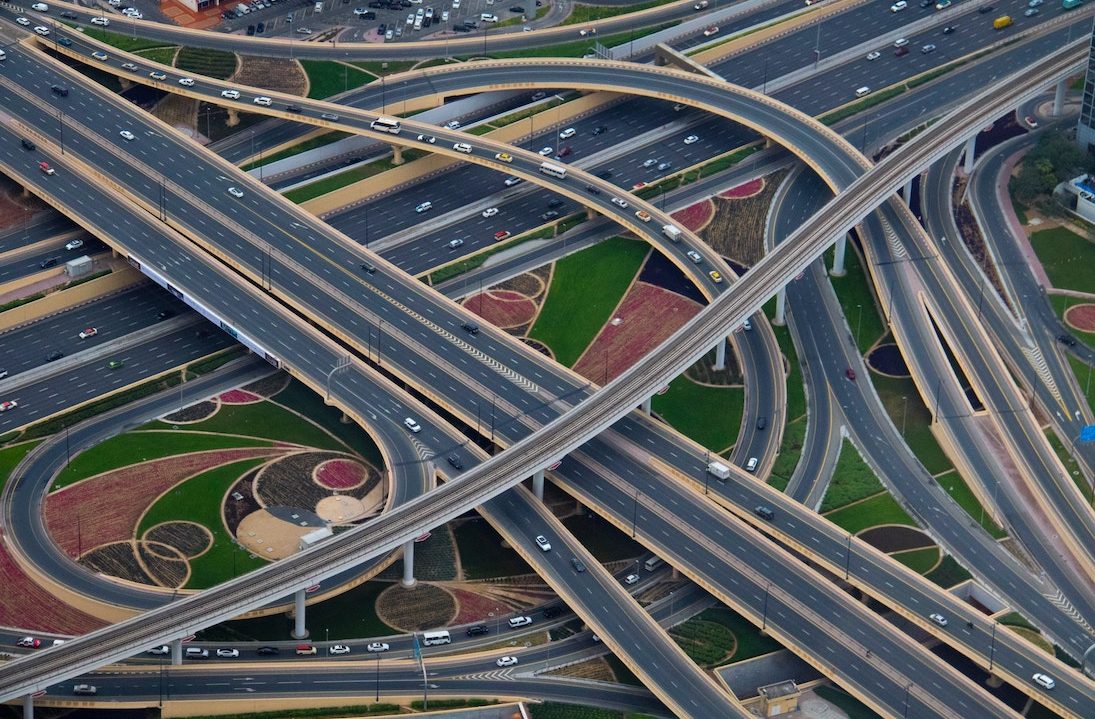
Lifting the Secrecy of Algorithms with Interpretability
As Artificial Intelligence and Machine Learning rapidly infiltrate critical areas of society, the lack of transparency surrounding these processes is becoming a tangible concern for the scientific community, the public authorities and the society at large. From financial and insurance markets to medicine, citizen security or the criminal justice system, the tendency has prevailed in recent years to devolve decision making to automated processing models in both public and private sectors. However, while able to outperform humans on many specific tasks, such models often operate as effective black-boxes, failing to provide a comprehensible account of how and, perhaps more worryingly, why they make their decisions. This situation demands immediate action from all parties involved to enforce a responsible and accountable use of machine learning and AI.
Deciphering the intricate mystery of what a neural network does to work out an output is most of the time an impossible feat that has to deal with complexity, secrecy, and lack of general rules and standards. The myriad of computations and distributed input-outputs across a number of layers makes the inner mechanisms of deep learning hard to understand, to say the least, when not completely opaque to human comprehension.
This opacity prevents companies, institutions and practitioners from fully understanding the models they deploy. Moreover, it precludes the identification and correction of biases and unfair practices. As Kate Crawford, principal researcher of Microsoft Research and founder of AI Now, puts it, “algorithms are only as good as the data they are trained with and data is often imperfect”. Algorithms that learn from labeled examples are susceptible to inheriting biases existing in the training data. Not in vain, examples in which machine learning models have been shown to reproduce existing patterns of discrimination are many and varied and could have severe implications.
As the justice system, the healthcare system or policymaking are increasingly intermediated by automated decisions, more and more voices have been raised to demand a strong approach to making this processes transparent, understandable and stripped of human biases or personal prejudices. Algorithms are used today to identify potentially dangerous individuals, decide who is being held without bail or distinguish between trusted and fake news in our social media feeds. The risk of making such decisions for reasons biased against sensitive attributes like gender, race or sexual orientation is too great to be overlooked. Hence, the need for understanding the data with which algorithms are trained and the logic they follow to discriminate among outputs is all the more evident insofar accountability is today, and perhaps more than ever, an ethical duty.
While many approaches to this issue are possible, understanding how models work is a necessary pre-condition towards a safe and fair automated processing. Thus the importance of machine learning interpretability, the scientific discipline that deals with explaining the processes that lead AI systems to make a certain decision. These processes are usually seen as “black-box”-es, in which inputs come in and outputs come out without a clear understanding of what happens between the two ends. Interpretability aims to shine light onto these “black-box”-es.
A research line of BBVA Data & Analytics is precisely working in a way of interpreting models that were not created ex profeso to be transparent, such as the aforementioned neural networks. The benefits of interpretability are clear: the ability to correct unfair outputs and to provide the data subjects with “meaningful information” that enables to vindicate their rights. Indeed, It is well worth remembering that the importance of making the right predictions is perhaps not as great as that of making sure that ”the right predictions are being achieved by the right reasons”.